ARCOL is a project funded by the French National Agency for Research (ANR) and coordinated by Baptiste Caramiaux, CNRS research scientist at ISIR, Sorbonne Université, in Paris.
The project began in early 2020. The project started on the idea to investigate interactive machine learning techniques to assist human learning in complex skills such as music performance or rehabilitation.
The first objective of the ARCOL project is to design machine learning strategies assist individuals in order to support the acquisition of skills.
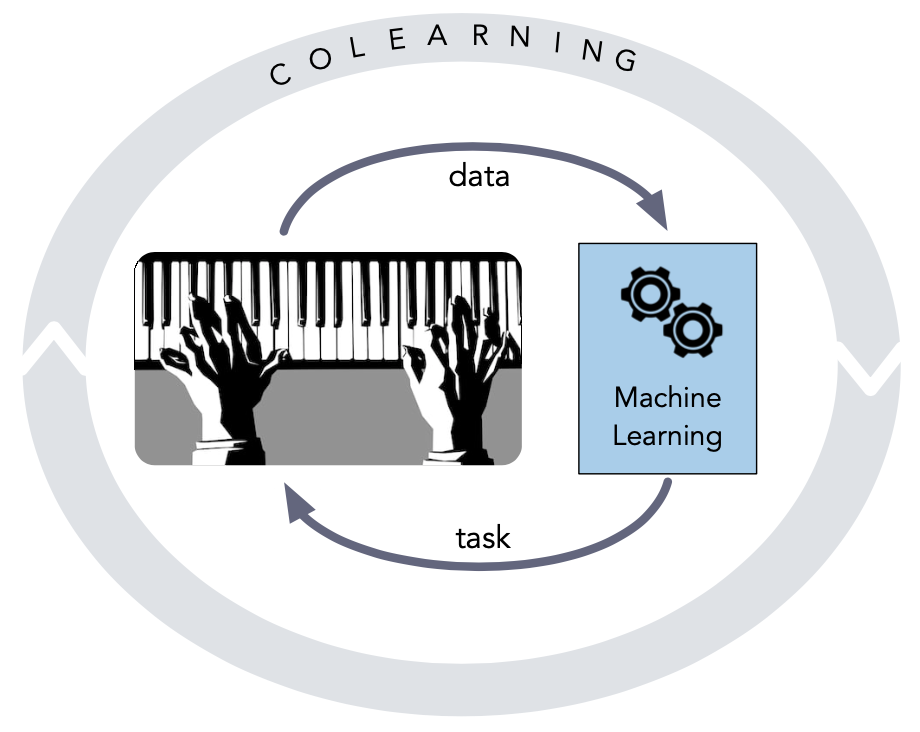
The COVID pandemic, which hit the world between 2020 and 2022, put the project on hold and led its members to rethink some of the objectives. Some solutionist ideas to solve the problem of technology-enhanced skill acquisition were challenged. Other more global issues, especially those related to the link between technology and society, emerged and became increasingly important to the team. In particular, we reflected on the cultural dimension of the technological object we are researching and developing.
There are scientific intentions in the technological approach to learning assistance that are driven by a techno-centric and progressive culture. This approach can lead to fundamental scientific results, as the project tries to show, but it also seems important to us to make this technological culture explicit. This means highlighting our positionality, as a researcher, towards our object of study, such as our subjectivity, the context of investigation and the time at which this investigation is conducted. But it’s also about seeing how artists and creators can bring alternative points of view to bear on technology, particularly machine learning and artificial intelligence, which are at the heart of the project.
From this, we try develop a methodology that is more inclusive from the point of view of the different actors in the project. A second objective of the project is therefore to study technological cultures, and in particular machine learning, and to explore methods that are more explicit about the underlying cultures of the stakeholders in the research process.
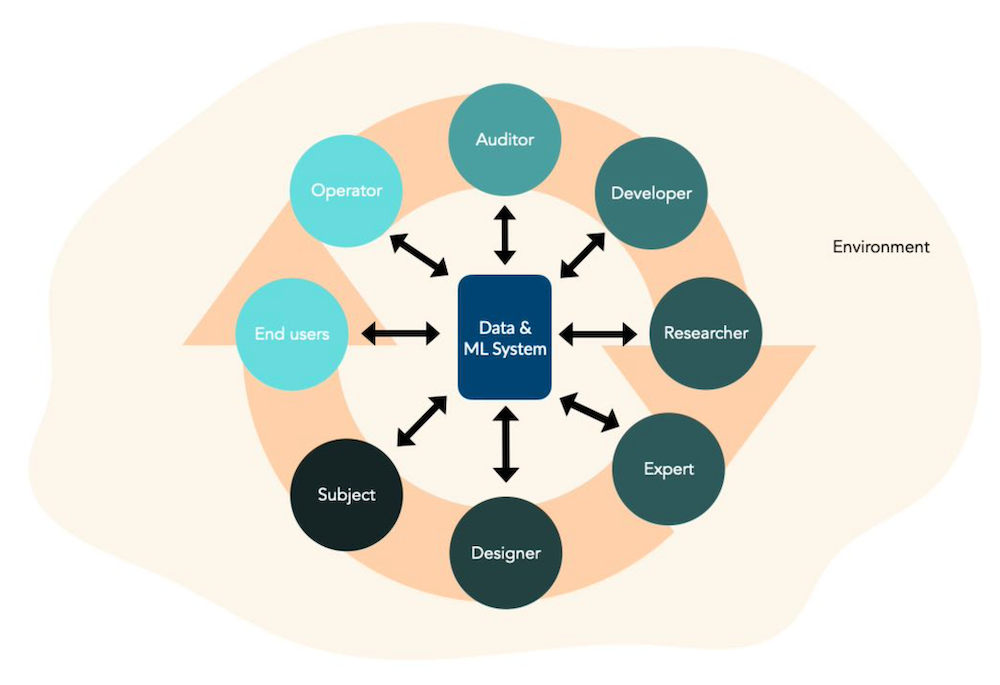
The project is supported by the ANR and CNRS, and conducted at ISIR (Insititute for intelligent systems and robotics) at Sorbonne Université.
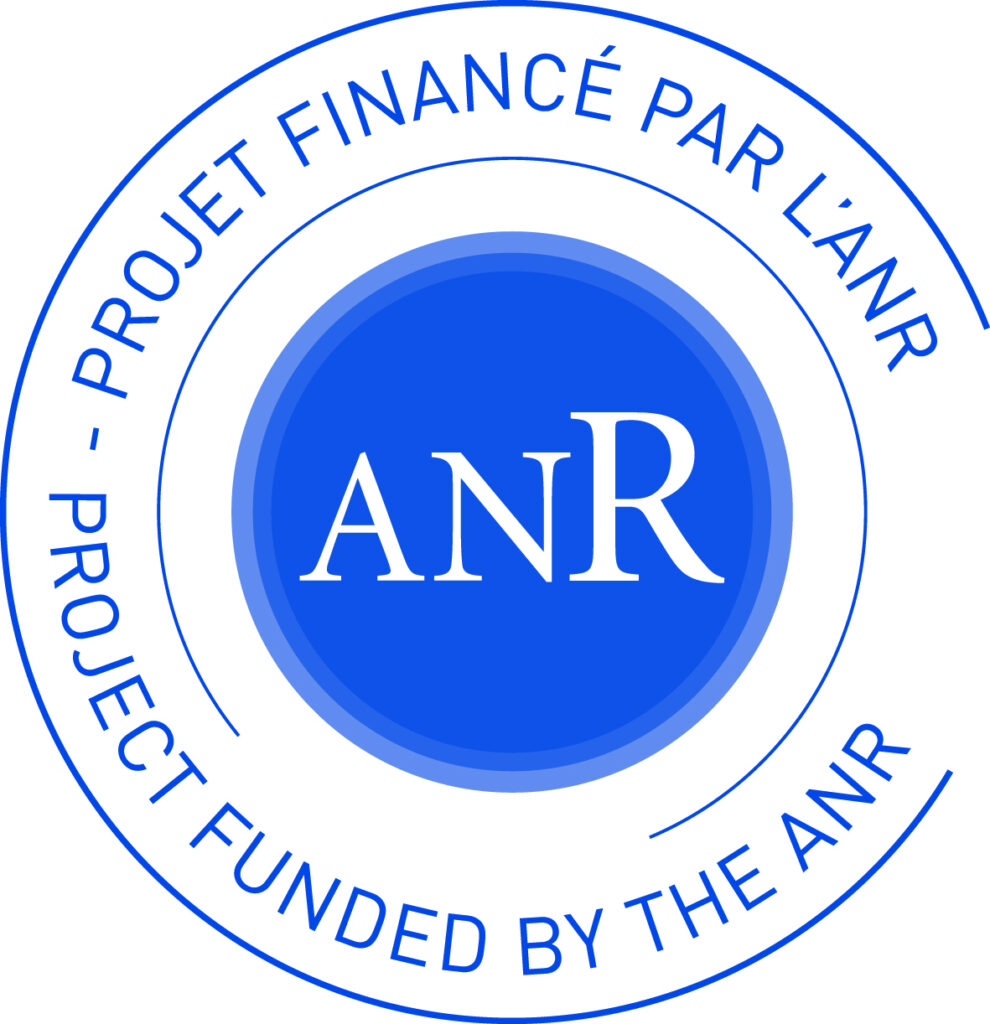
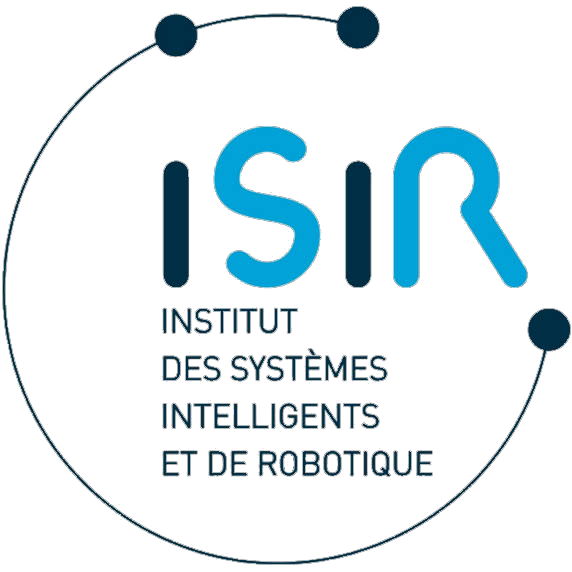
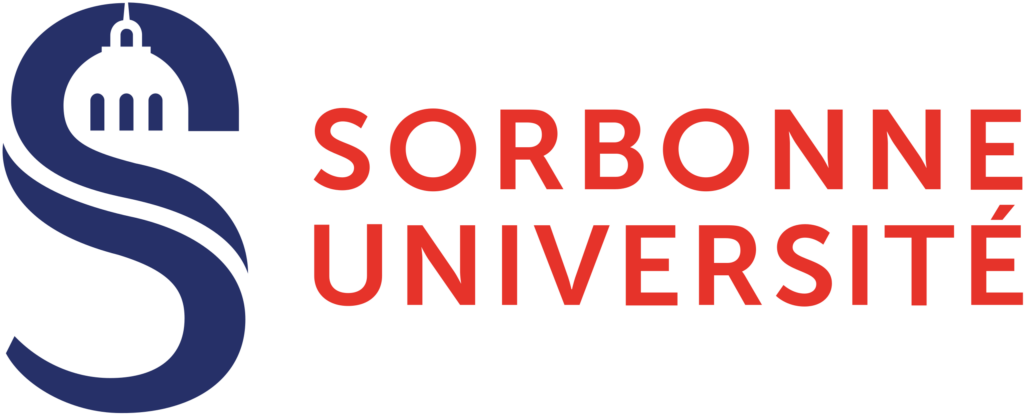