While hand gestures are intuitive for humans, it can be a difficult concept to convey to a machine. Given the increasingly complex functionality provided by modern prosthetic hands, machine learning-based pattern recognition of hand gestures has gained popularity over the last decade. In particular, it enables more complex movement through more degrees of freedom. However, this technology departs from traditional control strategies in that it relies on consistent and distinguishable muscle patterns, which are unique for each user. Previous research (e.g. Powell, 2014, de Montalivet, 2020) have shown the interest of training the user to enhance the co-adaptation process and improve accuracy. The training process also changes the mental model of the user, by reinforcing their understanding of how these prostheses work. We conjecture that the training strategy employed by the user can impact both accuracy performance and the mental model of the user. In this study, we investigate different strategies that can be leveraged by the user to teach a machine to classify eight hand gestures.
Link ↓ to view a video of the experiment.
Experiment_video.mp4 – Google Drive(opens in a new tab)
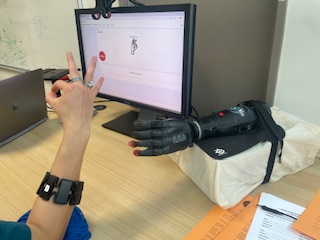